Not The Dreaded Doom
The first time I heard of and vaguely understood the phrase “machine learning” was roughly a year ago in a piece featured by Science Daily. I am loosely summarizing when I say that the point was that they were using images and computer algorithms to determine that it was time to harvest lettuce. This was mildly disappointing for me, as when I read “machine learning”, I found myself thinking of SkyNet from the titular film, “The Terminator”. However, the thought of computers being able to tell when it was time to cut that juicy piece of lettuce down on their own was equally fascinating.
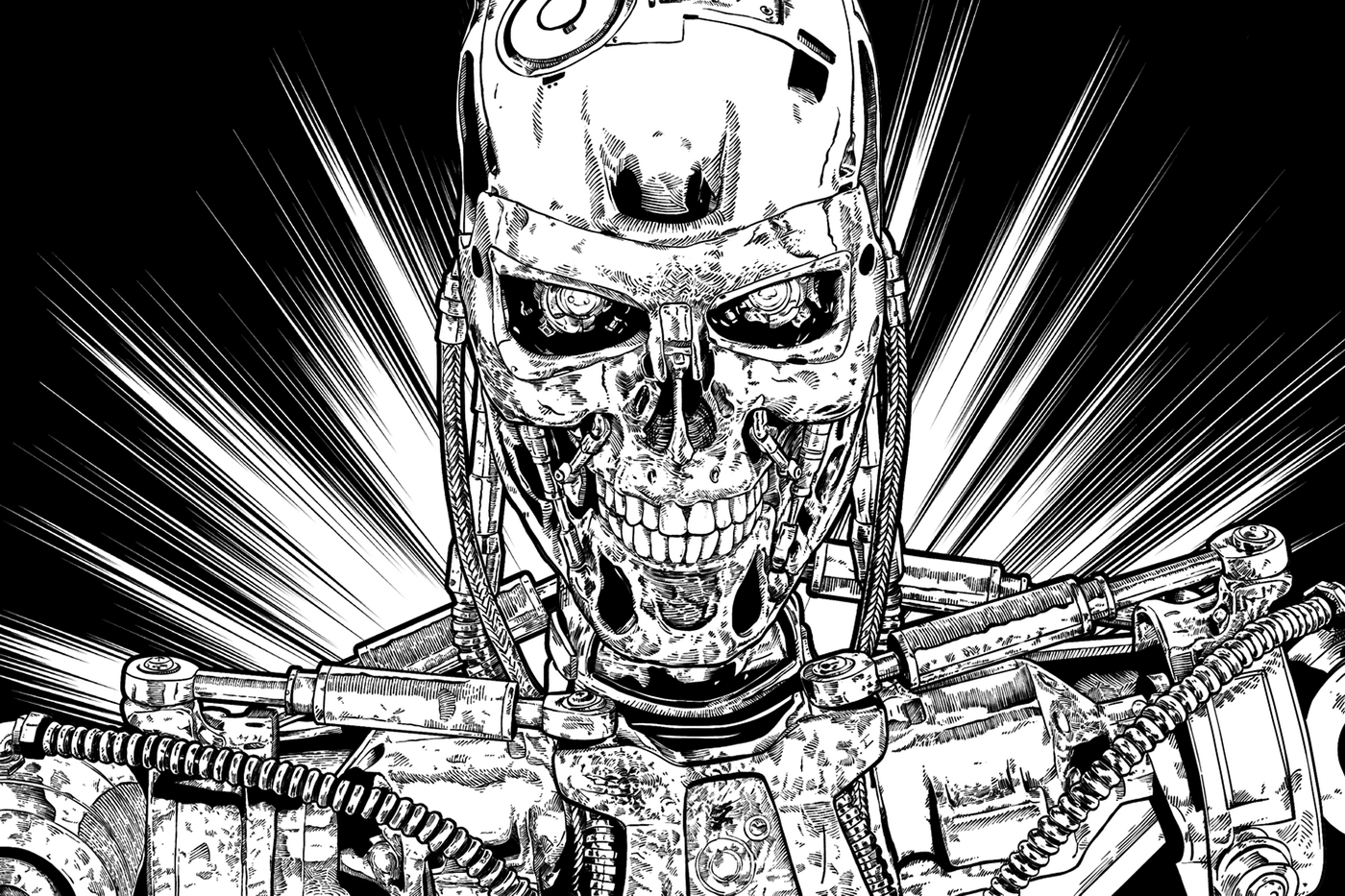
“Terminator again!” by Andy Veilski is licensed under CC BY-NC 4.0
In the present, I am wrapping up my first week with RET2020 and my lovely cohort, and these conversations on Machine Learning are just beginning.
I’m pulling lots of my definition from Karen Hao’s “What is Machine Learning?” (one of our assigned readings). Machine learning is essentially when the computer is able to predict, analyze, or create an outcome based on a series or subseries of data (can be images, numbers, etc). So far, I’ve begun to realize that this approach is being used in more places than I realized. Surprise to no one! It’s not just about lettuce.
Close to Home
Diabetes
The majority of the lectures that have taken place this week have been about using Machine Learning to address the issues of sociability, diabetes, and personalized devices. From Dr. Barua to Dr. Cote and Dr. Sabharwal, they have been providing us with their insights, wins, and hopes with regards to their amazing topics. Diabetes is something that needs no explanation but its impact in my family gives a certain heavier and more personal touch to this whole topic. If there is a way for these monitors and personal medical equipment to give us an insight as to where the patterns are with glucose levels and food consumption, then I’m all for it. Knowing is better than not knowing and if we can use machine learning to give us a more precise way of predicting trends by analyzing aggregates of data, I’m excited to see what else we can do.
And, of course, one thing that Dr. Barua mentioned in his presentation (not featured) was how the groups included pre-diabetic individuals in the study. A question I should have asked that didn’t occur to me at the time is, are there similarities between the Type 1 and pre-diabetic glucose trends after eating? And, if so, do you think we would see these trends in gestational diabetic patients?
I should also note that one of the pieces that stuck with me when Dr. Cote was presenting was his willingness to take feedback. I mentioned how a certain word choice might be better perceived by communities/groups if he made a simple substitution for a more relevant example. The feedback was well received and gives me hope that just as much as data matters, words continue to matter.
Autism Spectrum
One of the other readings (“Objective measurement of head movement differences in children with and without autism spectrum disorder“) that we had which also made me think about the people in my life was the research paper on head movement in AS and non-AS kids. This wasn’t addressed in a specific lecture but we had a conversation about this paper in my cohort. I’ve had a fair chunk of students who were Autistic and reading the paper made me think about the interactions that I have had with them: how they process stimuli, the head movements, all of it. The main wondering I have is how we can use machine learning to flag examples of sensory overload in order to better provide care for and educate those students. In the same way that I think people generally don’t understand Diabetes, I don’t think people have a good understanding as to what Autism is and how it can look in kids (and even adults). I want to be clear, I don’t want to “fix” Autistic individuals but I do want to fix the fact that society tends to not understand or know how to approach them.
So, About My RET 2020: Rice Cohort
I have greatly enjoyed this group of people who are bringing this wide berth of experiences into our midst. I have already tweeted out some great one liners, especially one from Chris, which have made me chuckle all day. There is so much knowledge in this group that I wish I could just soak up but there is also so much love. It’s clear to me that this group of amazing educators not only values the lives and future livelihood of their students but also has an inherent dedication to building that better tomorrow today.
I’ve had more laughs and genuine human interactions on Zoom calls this whole week than I’ve had the past 3 months, so I greatly appreciate that. It is my hope that we continue to grow and bond and work towards our goals. But, I also hope that we can take what we have learned here and take it back to our kids and spread that educational wealth. Without getting too sentimental, this work is important and feels even more relevant with this fantastic group of people around me. There seems to be too much dark in the world with COVID, blatant police brutality, and of course the unfettered racism, it feels good to finally be able to snatch and hold onto some light.
One Response to Another Teacher COVID Story: Ch.1 Learning of Machine Learning